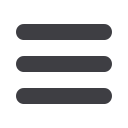

臺大管理論叢
第
27
卷第
3
期
97
The Impact of Multicollinearity on the Evaluations of Regressors:
Comparisons of Effect Size Index, Dominance Analysis and
Relative Weight Analysis in Multiple Regression
1. Purpose
Regression analysis is frequently used in the social sciences (Aguinis et al., 2009;
Cascio and Aguinis, 2008; Casper et al., 2007). The purpose of this paper is to review the
properties of the effect size index and the measures of relative importance derived from the
relative weight analysis (RWA) and the dominance analysis (DA) that are used to evaluate
the predictors under multicollinearity. The general purpose of multiple regressions is to learn
about the relationship between several predictors (i.e., regressors) and a criterion variable.
Regression analyses, however, often rely heavily on hypothesis testing and interpretations of
regression coefficients and ignore the effect sizes of regression models and the qualities of
individual predictors (Courville and Thompson, 2001; Kelley and Preacher, 2012; Nimon
and Oswald, 2013). This issue is particularly important when there exists multicollinearity
among the regressors/predictors. The focus of this paper is on the performances of the
relevant statistics from the RWA and the DA, as well as several index of effect sizes, under
three effects of multicollinearity (enhancement, suppression, and redundancy) (Friedman and
Wall, 2005).
In this paper, the following effect size index are considered: zero/partial/semi-partial
coefficients, structural correlations, regression coefficient-based statistics, and product
measures. On evaluating the relative importance of the predictors, the RWA creates the
relative importance weights (RIW) (Tonidandel et al., 2009) that addresses the properties of
correlated predictors by creating the orthogonal counterparts of the original predictors. On
the other hand, the DA creates the Dg coefficient that can reflect the relative importance of
predictors (Azen and Budescu, 2003; Budescu, 1993). Based on the examination of the R
2
values for all possible subset models, the DA generates the D
g
coefficient and two different
measures of dominance that differ in the strictness of the dominance definition (the
conditional dominance and the complete dominance). Compared to the traditional
correlation-based and regression-based coefficients, the RIW and the D
g
coefficient are more
intuitive, meaningful, and informative measures that can indicate the importance of
predictors. In this paper, a simulation and a survey data analysis are used to demonstrate the
performances of these index statistics under multicollinearity.
Haw-Jeng Chiou
, Professor, Department of Business Administration, National Taiwan Normal University