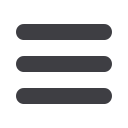
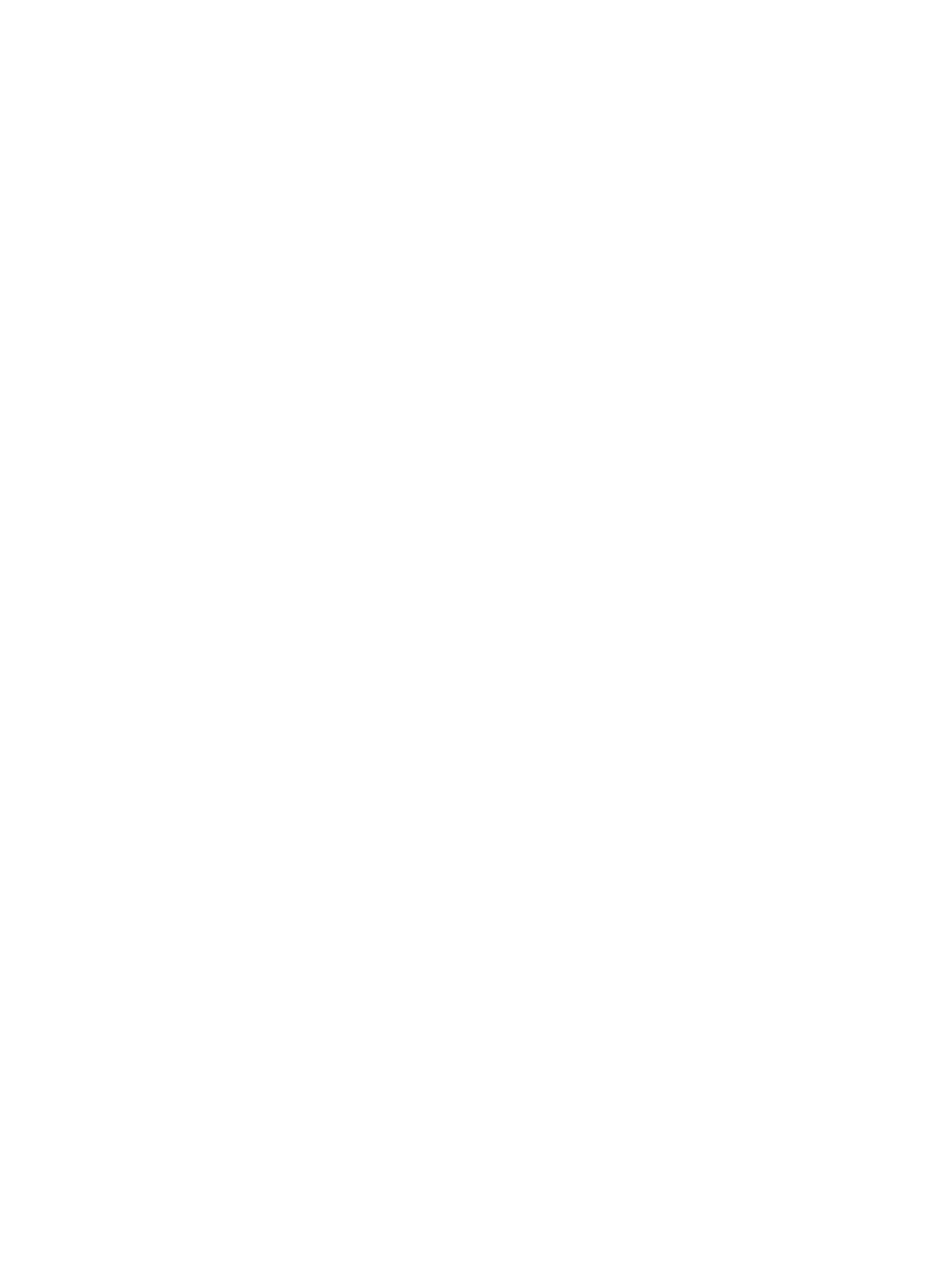
臺大管理論叢
第
27
卷第
3
期
99
All index as well as the RIW and the D
g
coefficient are computed by R software (R
Development Core Team, 2014) and the 95% confidence intervals for statistical inference are
constructed by bootstrapping with bias corrected accelerated (BCa) method (Canty and
Ripley, 2014).
3. Findings
The simulation results show that without multicollinearity, all the index statistics can
reflect the relative importance of the Predictors. When multicollinearity presents, however,
only the RIW and the D
g
coefficient can consistently indicate the relative importance of the
predictors. Index such as the product coefficients fail to reflect the expected order of
importance due to the inconsistent directions of the correlation coefficients and the
regression coefficients.
The results from the survey data reveal that, regardless of the use of the index statistics,
the years of formal education is the strongest predictor of salary. However, due to
multicollinearity, different statistics indicate the importance of the predictors in different
ways. Similar to the results from the simulation study, both the RWA and the DA show a
stable function for evaluating the relative importance of the predictors. In particular, the DA
has the advantage of flexible procedures for evaluating the different facets of dominance of
the predictors.
4. Research Implications
This paper demonstrates the substantive differences of several index statistics for
evaluating the relative importance of predictors in multiple regressions. Several implications
can be mentioned. First of all, applications of regression analysis have to consider both the
statistical significance and the practical significance of the overall model (Cohen et al., 2003;
Pedhazur, 1997). A significant R
2
has to be established to support a regression model, and
then the explanations of individual predictors can follow. Secondly, researchers have to
clarify the purpose of the index statistics for evaluating the individual predictors or the
relative importance (Budescu, 1993; Johnson, 2000; Johnson and LeBreton, 2004). The
former could be made by many traditional correlation and/or regression coefficients. The
latter, however, can only be done by a limited set of statistics, namely the RIW and the D
g
coefficient. This is particularly true in the presence of multicollinearity.