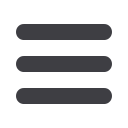
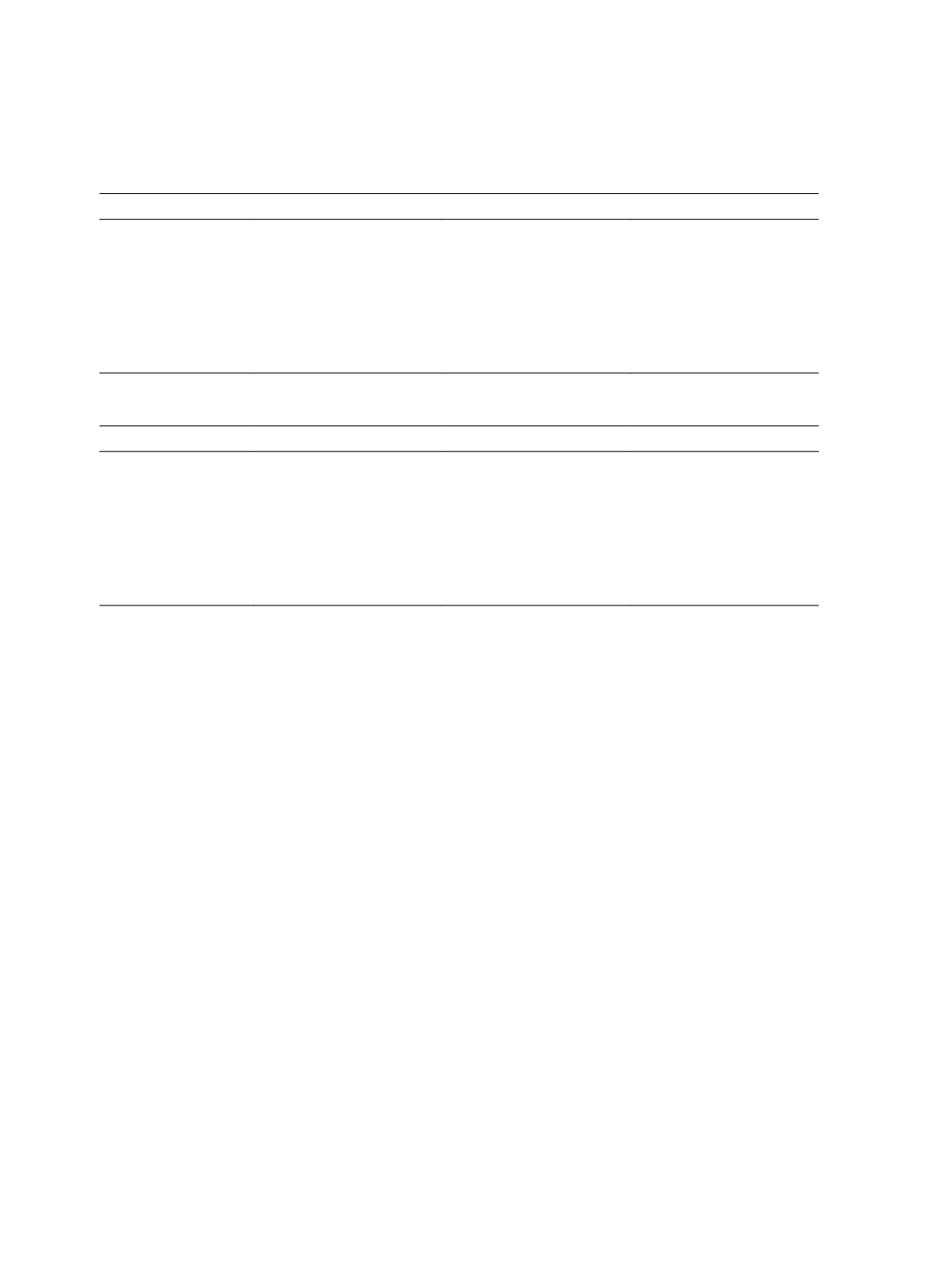
臺大管理論叢
第
27
卷第
2S
期
55
Table 10 The Comparison on the DY2009 Dataset
SFC algorithm
SFC (worst)
MFU2Ps
#FU2Ps
3856
3856
3856
Total distance
60.55
195.70
1201.73
#Clusters
43
2731
3572
Quality index
2603.65
534444.57
4292579.56
Runtime
1
(s)
480.85
480.85
486.77
Runtime
2
(s)
3.51
1.93
-
Table 11 The Comparison on the AirQuality Dataset
SFC algorithm
SFC (worst)
MFU2Ps
#FU2Ps
5969
5969
5969
Total distance
52.61
265.50
2379.21
#Clusters
81
3015
4710
Quality index
4261.41
800479.83
11206079.10
Runtime
1
(s)
426.47
426.47
428.67
Runtime
2
(s)
7.46
3.81
-
5. Conclusion and Future Work
In big data analytics, the presentation of the mining result is an important issue. For data
that is composed of univariate uncertain attributes, mining frequent univariate uncertain
patterns (FU2Ps) is an effective way to understand the nature of the data. However, the
number of FU2Ps is usually too large to be comprehended by users. In this study, we propose
the SFC algorithm for summarizing FU2Ps. The SFC algorithm summarizes a set of FU2Ps
by representative FU2Ps and statistics of the FU2Ps belonging to each representative FU2P.
The hierarchical clustering technique is adopted to retrieve the summary. Instead of
examining a large number of FU2Ps, users only need to check tens of or perhaps hundreds of
representative FU2Ps. This greatly improves the practicability of FU2Ps.
In the future, we plan to investigate the possibility of further merging. The merging
effect is not very obvious in the experiments. To provide a more concise and still meaningful
representation, we will develop auxiliary or new methods for merging. We also try to find or
develop more suitable clustering techniques.