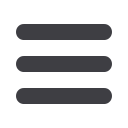
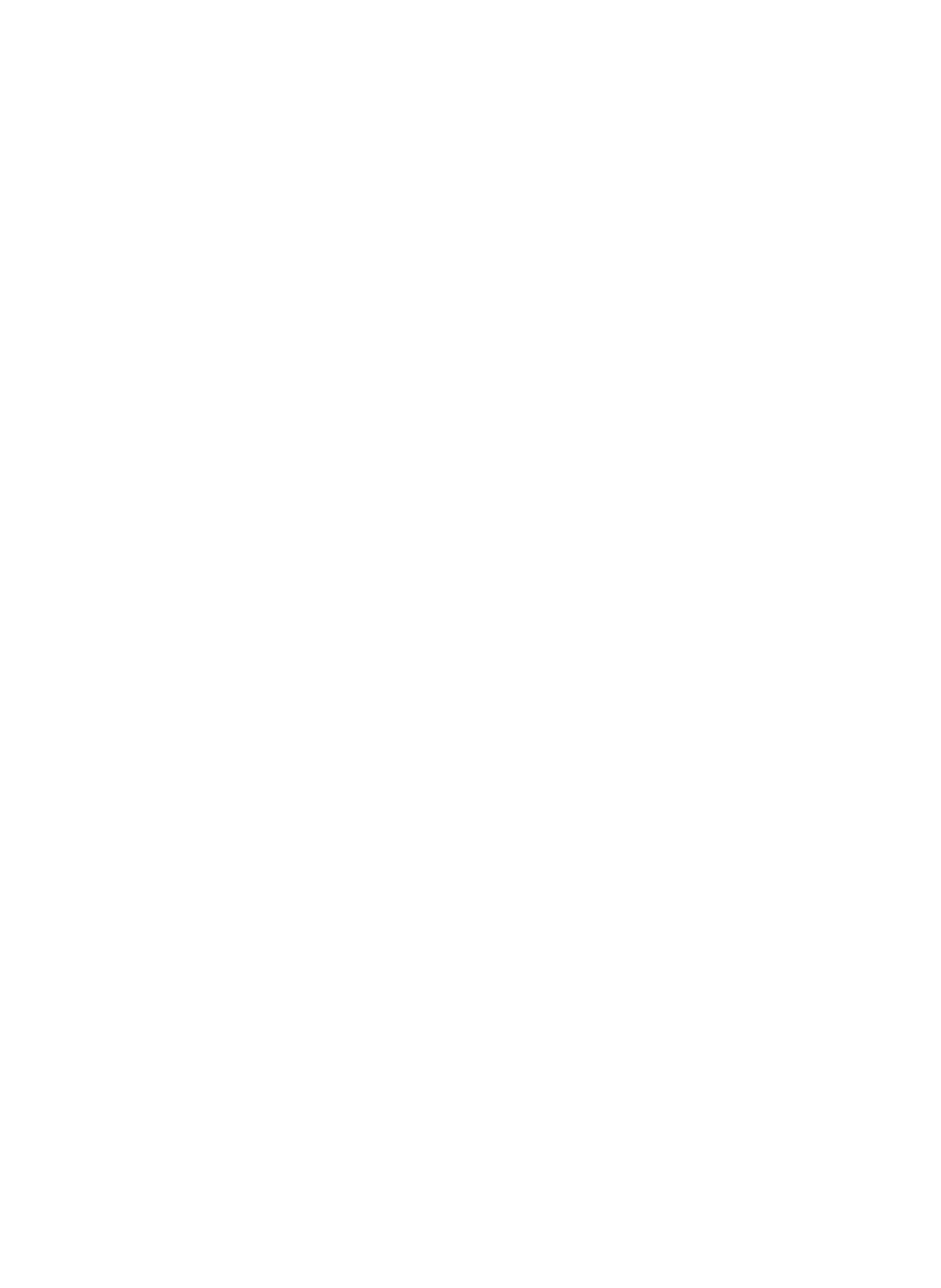
臺大管理論叢
第
27
卷第
2S
期
53
The runtimes required for the three datasets do not change significantly under most
parameter settings. In both real datasets, runtime significantly increases when
w
equals one.
The increases in runtime result from longer clustering processes.
The number of clusters increases as
ξ
becomes larger. In the synthetic dataset, the
number of clusters is significantly high when
w
equals one. It is because the FU2Ps of the
synthetic dataset have a wide range of expected supports. Instead, the number of clusters is
significantly high when
w
equals zero for the both real datasets provided that the FU2Ps of
each real dataset have more different appearance.
To sum up, we should consider both appearance and expected support in distance
function at the same time, only considering one may result in bad summarization quality. For
the synthetic dataset, using a lower
ξ
and a higher
w
results in better summarization quality.
For the both real datasets, only the zero
w
leads to bad summarization quality. Therefore, we
suggest to use a relatively higher
w
in the SFC algorithm since the experiments with higher
w
have good summarization quality. The value of
ξ
influences the number of derived
clusters. Users can choose a suitable
ξ
according to the number of representative FU2Ps they
wish to have.
The generated summaries can represent a set of FU2Ps well. For example, a
representative FU2P in the DY2009 dataset is [temperature 26°C to 30°C, relative humidity
67% to 76%], whose expected support is 312.95. This representative FU2P represents 94
FU2Ps, most of which have similar appearances and expected supports, such as [temperature
27°C to 30°C, relative humidity 69% to 77%], [temperature 27°C to 31°C, relative humidity
68% to 75%], [temperature 26°C to 30°C, relative humidity 73% to 80%], and [temperature
26°C to 29°C, relative humidity 71% to 80%]. The lower bound and upper bound of the
expected supports in this cluster are 310.21 and 315.29. Both bounds are close to the
expected support of the representative FU2P.
In the two real datasets, most of the representative FU2Ps are composed of two or three
attributes. For the DY2009 datasets, most of the representative FU2Ps comprise temperature
and relative humidity. This indicates that temperature and relative humidity are highly
related and have some frequently occurring combinations in Taiwan. For the AirQuality
dataset, the attributes that comprise the representative FU2Ps are more varied.
4.3 The Comparison with MFU2Ps
The set of MFU2Ps is a form of summarization. A MFU2P can be treated as a cluster
medoid and the FU2Ps which have this MFU2P as the closest medoid are the cluster