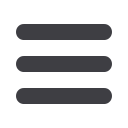
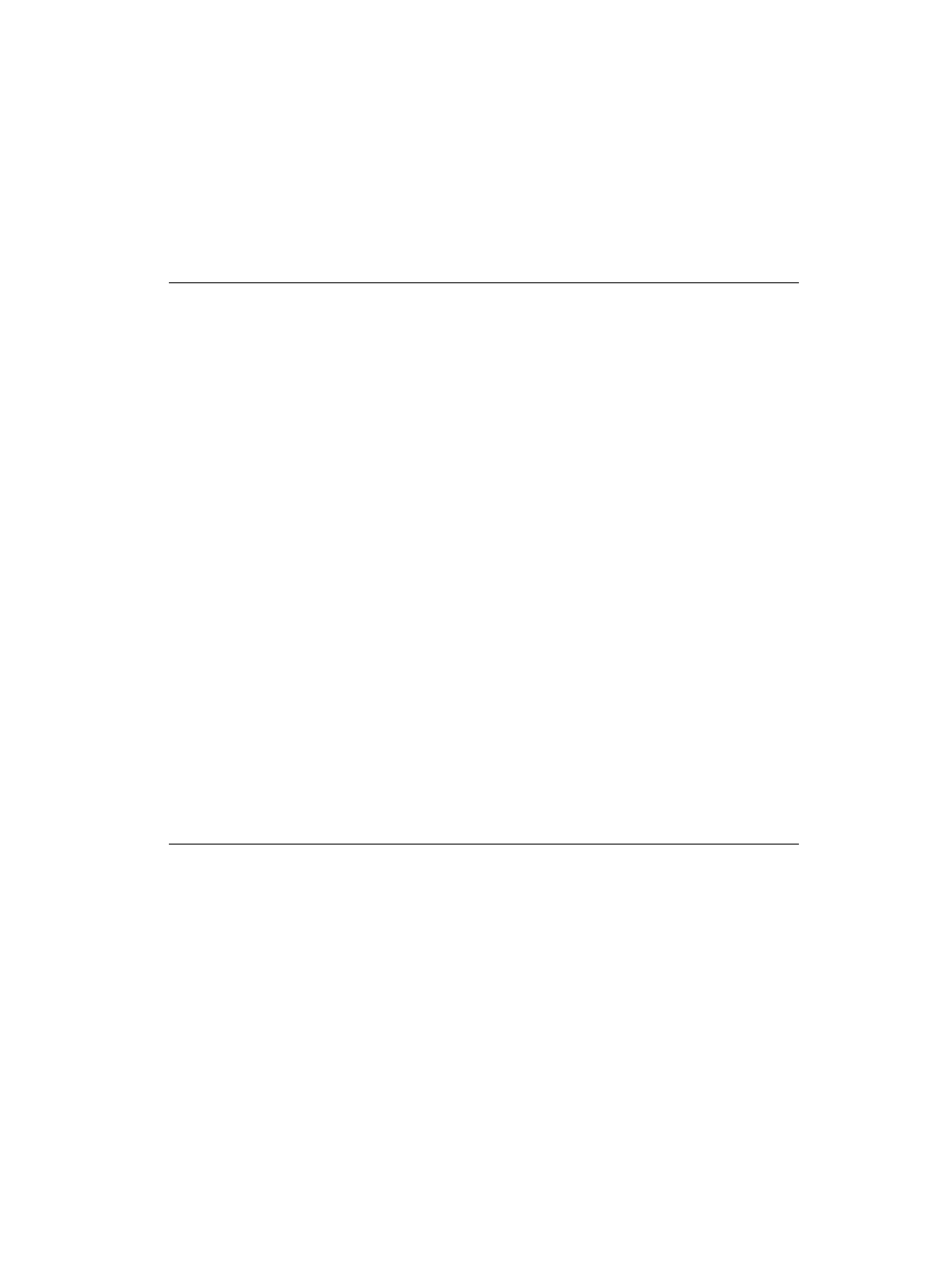
為頻繁單變量不確定樣式產生摘要
44
In the SFC algorithm, the FU2Ps are treated as the objects in Figure 2. The returned
medoids are the representative FU2Ps. We will refine the set of representative FU2Ps in the
next step.
Algorithm:
modified k-medoid
Input:
An initial set of medoids
A set of
n
objects
Output:
A set of updated medoids
1.
repeat
2. Let
Changed
be false;
3.
for each
cluster
C
i
do
4. Find the object o in
C
i
, which has the smallest average distance
5. between itself and other objects in
C
i
;
6.
if
o
is not the medoid of
C
i
then
7. Set the medoid of
C
i
to
o
;
8. Set
Changed
to true;
9.
end if
10.
end for
11.
if
Changed
is false
then
12. Return the current set of medoids;
13.
end if
14.
for each
object
j
do
15. Find
j
's nearest medoid, and assign
j
to the cluster of this medoid;
16.
end for
17.
end repeat
Figure 2 The Modified
k
-medoids Algorithm
3.3.3 Steps 3 and 4: Deal with the Unsatisfied Clusters
In the previous step, the SFC algorithm derives a set of clusters. We refine this result by
searching each cluster for FU2Ps that are not well-assigned. We use a quantitative measure
“silhouette” (Rousseeuw, 1987) to determine whether a FU2P is well-assigned or not. For
each FU2P
c
, let
a
(
i
) be the average of the distances between
i
and all other FU2Ps in the
same cluster. For each of the other clusters, we also compute the average of the distances
between
i
and all the FU2Ps in this cluster. Let
b
(
i
) be the lowest such average distance.
Silhouette of i is defined as follows: