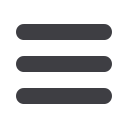
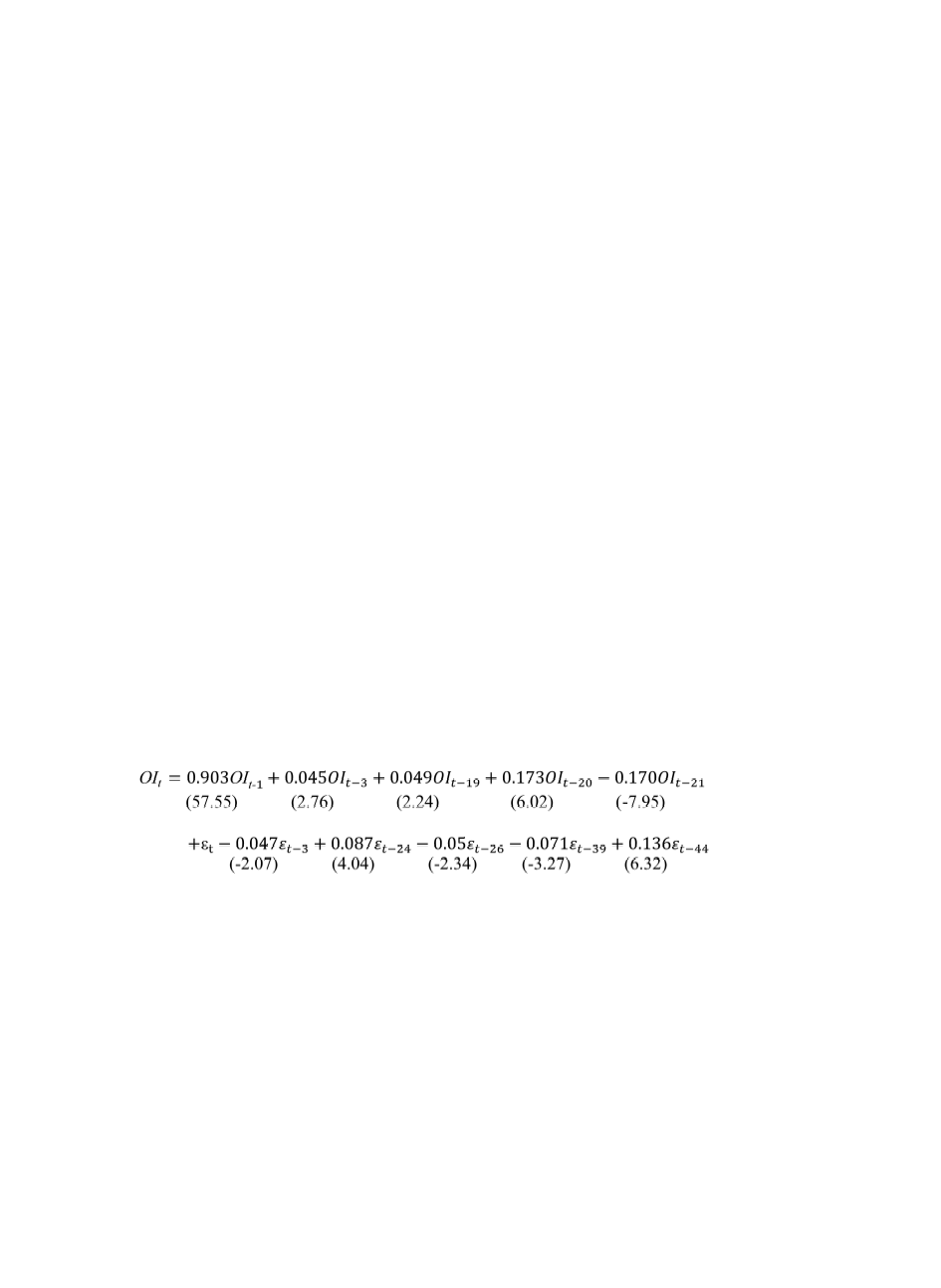
臺大管理論叢
第
26
卷第
3
期
181
Appendix A
We estimate the Box-Jenkins ARIMA model suggested by Bessembinder and Seguin
(1993) to partition open interest into expected and unexpected components. The three phases
of the ARIMA (
p,d,q
) are identification, estimation and diagnosis, in that order. First, if the
data explodes over time, it needs to be differenced
d
times to achieve stationary value by the
unit root tests. In our result, the OI variable is a stationary
I(0)
time series. We then utilize
the correlogram including the autocorrelation function (ACF) and the partial autocorrelation
function (PACF) to decide the order (
p
and
q
) of the autoregressive, or AR, term and the
moving average, or MA, term. Second, we estimate the candidate ARIMA specification to
obtain the all parameters of the ARIMA model. The non-significant intercept and coefficients
are omitted for a parsimonious principle and then repeat the second step again. Third, we
make sure that there is no serial correlation in the residuals, using the Ljung-Box Q statistics
test for diagnostic checks. The null hypothesis is that there is no serial correlation in the
residuals up to the specified order. If the result rejects the null hypothesis, we should return
to the first and second steps. We also use AIC (Akaike, 1974), SBC (Schwarz, 1978) and
extended ACF (Tsay and Tiao, 1984) to verify the chosen number of lags. Following the
above steps, the lowest AIC and SBC values are 19.31195 and 19.33779 respectively. The
final model used to decompose the OI series is ARIMA [(1,3,19,20,21), 0, (3,24,26,39,44)]
with parameters as follows:
Numbers in parentheses are t-statistics. All coefficients are significant at the 5% level.
The ACF and PACF of residuals at least 60 lags are nearly zero and their Ljung-Box Q
statistic test with large p-values, suggesting that the null hypothesis of no autocorrelation in
the residual series is not rejected at a 5% significant level. The results of extended ACF are
similar to the above results.