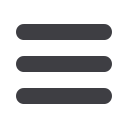
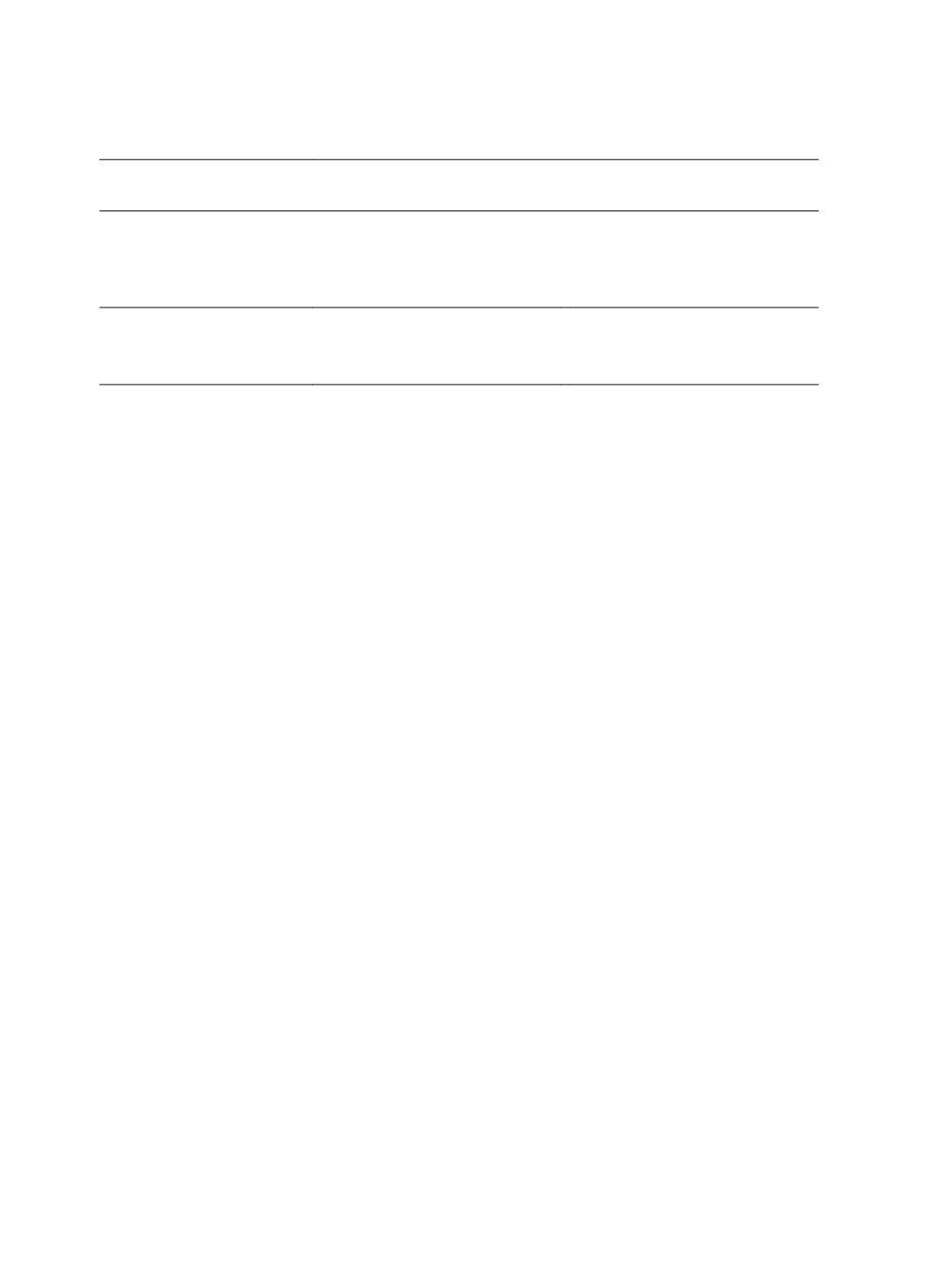
臺大管理論叢
第
26
卷第
3
期
173
Model (7)
Model (8)
VOL
t
|BDEP
t
– ADEP
t
|
Thursday (
κ
3
)
350
(0.19)
3.7111
b
(2.39)
Friday (
κ
4
)
1,270
(0.73)
3.4306
b
(2.30)
H
0
:
β
1
=
β
2
54.42
a
2.89
c
H
0
: all
κ
= 0
2.23
b
1.94
Adj. R
2
0.5696
0.1084
Note: Regressions (7) and (8) test the implications of Hypothesis 3. The dependent variables are
volume (
VOL
t
), and the absolute difference between bid and asked depths (|
BDEP
t
– ADEP
t
|),
respectively. |
ΔOI
t
| is the unsigned change in open interest.
DiOI
i
(
I
= 1, 2) are dummy variables
representing the increment or decrement of open interest.
DiOI
1
= 1 when
ΔOI
> 0, and zero
otherwise;
DiOI
2
= 1 when
ΔOI
< 0, and zero otherwise.
EOI
t
, (expected open interest) and
UOI
t
(unexpected open interest) are partitioned from
OI
t
using ARIMA model.
TTM
t
is the time-to-
maturity (in days) of the highest open interest futures contracts.
D
j
(
j
= 1, 2, 3, 4) are the four
daily dummies for the day-of-the-week effect. Numbers in parentheses for regression coefficients
are t-values. H
0
:
β
1
=
β
2
is the joint test (F-statistics) for whether the effects of increments and
decrements in open interest are the same. The significance of the day-of-the-week effect is
jointly tested by H
0
: all
κ
= 0, also reporting the F-statistics. The superscript a, b and c indicate
significance at the 1%, 5% and 10% confidence levels, respectively.
4.5 Robustness Analysis
Note that the process detailed in section 3.1 for decomposing the OI is critical to this
study. Because the empirical results are based on the decomposition of open interest, the
model for decomposition should be chosen with caution. In this section, we explain the
reasons of our preference for a more precise model over other parsimonious models, and
discuss the robustness of our main findings using alternative decomposition models. Table 7
presents seven alternative ARIMA models and their time series properties, along with the
final model (Model (8) with bold text) used in the study for the OI decomposition. The final
model has a few advantages over its parsimonious alternatives. First, the residuals from our
ARIMA model are nearly white noise series. There is no serial correlation up to 60 lags.
Second, outliers should be controlled in the univariate model. Third, the model has very
small AIC and SBC among other more parsimonious specifications. For the three models
(Model (5), (6) and (7) in italic) with even smaller AIC and SBC, the residuals are not white
noise according to the Q-statistics. Models with relatively parsimonious form (e.g., Model
(1) to (4)) clearly is not econometrically appealing, if judged by the AIC and SBC.
Two disadvantages associated with a more complicated model are the loss of degree-of-
freedom and the difficulty in interpretation. The loss of degree-of-freedom should not pose